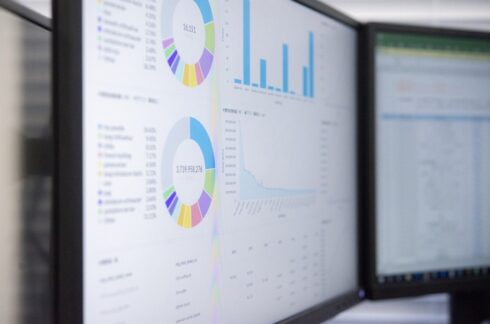
Organizations now are looking towards AI intelligence to help operators avoid manual operations, whether it’s due to the sheer number of alerts that they’re getting that are contributing to “alert fatigue” or the lack of observability of how the organization is working.
HCL has introduced a new set of features to its HCL Workload Automation Platform (HWA) version 10, providing IT and business automation with streamlined modeling, advanced AI, and open integration for observability through the integration of AI.
HWA 10 introduces AI-powered anomaly detection for workloads and also includes observability through open standards to monitor cloud-native environments.
The improved observability that operators gain comes down to three main telemetries: Metrics, Logs, and Traces.
“This is basically the idea of giving our customers the possibility to monitor the product together in an easy way with all the rest of the cloud environment, getting all the data they need from our product,” Marco Cardelli, the Lead Product Manager at HCL Workload Automation.
AI features to enhance observability
AI is a core feature of any observability initiative as it can provide anomaly detection and the identification of patterns. It can also help reduce the volume of alerts and messages that often cause operators fatigue. The use of AI can also help with job issues that stem from root-cause analysis and can improve the user effectiveness of DWC (Dynamic Workload Console).
Meanwhile, the new AI Data Advisory component in Workload Automation is a core feature that is available on containers.
“The component ensures that the operators can get early detection of workload execution issues. This is achieved through big data machine learning analytics technologies applied to the data from Workload Automation,” Cardelli said.
Observability not just for power users
The operators need to always know how their workload execution is going without the need for power operators.
“Currently, what happens is that normally, there are what we call power operators. So operators have a long experience with the product that is able to detect the intuitive way, it’s something that is going wrong, they know how it normally goes,” Cardelli said.
The HWA platform hopes to give that level of observability to more than just power operators.
After running the AI Data Advisory, the workload automation data is then published through OpenMetrics, after which the AI can then periodically read the data, predict trends, and detect any anomalies. If there are any anomalies detected, the tool will then send an alert to the HWA UI.
The tool can compare the execution of a day’s workload and compare it with a similar execution day to try to detect if there are any things that are going on differently.
Cardelli added that observability through the use of AI/ML opens up a lot more possibilities for how customers can analyze their data.
“Now [users] have dashboards on the user interface on the dynamic workload console where they can monitor the execution of the workloads. They can define widgets for lists of jobs that have errors so they can really monitor the statistics of the overall current workload execution,” Cardelli said. “Rather than rely on power operators, we want to allow our customers to have an AI-powered way to detect these kinds of anomalies.”
HWA can also help operators stay on track by providing the workload service assurance feature that is able to detect if one is missing any SLAs during the final deadline of some of the most critical jobs.
The open standards for HWA data collection can now be integrated with some of the biggest observability tools in the market including Grafana, Prometheus, Instana, and many more. Predefined dashboards will be provided as an add-on for Splunk and Dynatrace.
Start your 90-day free trial and get hands-on experience with a one-stop automation platform, click here.
Content provided by ITOps Times and HCL Software.