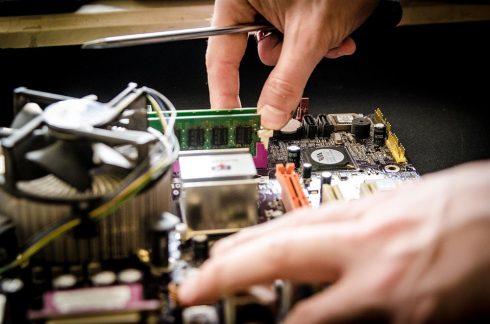
The explosion in operational data and machine-learning compute capacity is finally enabling AIOps — Artificial Intelligence for Operations. But like many other technologies, AIOps fits within a larger organizational and systems context, and enterprises need to ensure that they are ready for the shift.
Successfully implementing an AIOps solution requires an awareness of the potential problems associated with such a transition. Here are four key challenges that organizations will face as they look to adopt an AIOps solution, and how to address these risks:
Challenge 1: Identifying Use Cases (not just processes)
Companies that don’t identify the underlying issues they’re trying to address with AIOps tend to utilize an incremental approach. Each new AI- and ML-related feature may seem like an easy way to increase efficiency – replacing an existing sub-process with supervised learning, for example.
However, the most meaningful results will come from rethinking operational use cases in a top-down manner to complement these bottom-up process improvements. AI/ML algorithms have very different strengths and weaknesses than human operators, meaning that the maximum value of the algorithm is realized in workflows that are different from those that were created for humans. For example, self-driving cars don’t limit themselves to placing two cameras in the driver’s seat to mimic human eyes, nor did they force the cameras to swivel around like a human neck. Rather, these cars can utilize a half-dozen fixed cameras all around the car and supplement this data with LIDAR, RADAR and even ultrasonic sensors.
The same notion applies to AIOps. Data and processes that have been optimized for humans may not be the best way to leverage these algorithms. To avoid an inefficient piecemeal adoption lifecycle, enterprises should start with a top-down assessment of all the systems, applications and processes to determine where integration of AIOps might have the most impact.
RELATED CONTENT: Why automated AIOps is better than AIOps
Challenge 2: Not Enough Data
Even the most powerful AIOps tools can be impaired if they don’t have enough data to process. AI/ML algorithms are famously data-hungry, requiring both a large training data set as well as ongoing real-time data for robust inference.
A first step in preparation for the AIOps investment is to implement a performance measurement system that looks across all layers of the app code, hardware and software infrastructure, and even user and business data. This initiative will both provide the company with greater visibility for current operations and also build the right platform for an effective AIOps implementation.
Challenge 3: Low-Quality Data
Once there’s a process in place to collect an adequate volume of data, the next step is to assess its quality. Common problems that we see in the field today include noisy data, inconsistent or insufficient reporting frequencies, and even inconsistent naming policies across applications or datacenters.
Organizations should develop procedures for standardizing and filtering data collection, as well as identifying the types of data that are most valuable for their specific priorities. Adopting procedures based on this shared understanding will provide value now and in the future.
Challenge 4: Meaning of Data
Companies can collect abundant amounts of high-quality data, but without the right context, the data is nearly useless. Data points that lack semantic definition/consistency are less valuable for both human operators and AIOps – for example, do the values of a given metric like “user transaction rate” represent a per-second, per-minute, or per-hour rate? And was the choice made here applied consistently across other related metrics?
As another example, if data is being collected from a microservice that’s running within a container that’s running on a VM that’s running on a physical host, is the data tagged at all levels so that behavior at one level can be correlated and compared to other levels? Otherwise, enterprise data can resemble a series of siloed, parallel universes.
To address this issue, teams should emphasize the importance of linking data so that relationships are recorded and easily identifiable. A standardized naming policy can help keep companies from forking off new silos of data.
Maximize the Investment
AIOps promises to tackle some of the toughest IT issues, but there are core organizational issues that teams will need to address before they can realize that value.
Because some of the most common problems organizations encounter when it comes to AIOps integration are found in the quantity, quality, and interpretation of their data, the first critical step – before jumping into an AIOps solution – is to take a meaningful assessment of existing IT systems and use cases. That includes the collection methods, business motivations, and contextual meaning behind the data.
By building a foundation of robust data infrastructure and clear use case identification, companies will find that their future AIOps investment will not only deliver what it promised but also provide ongoing additional value that wasn’t even expected.