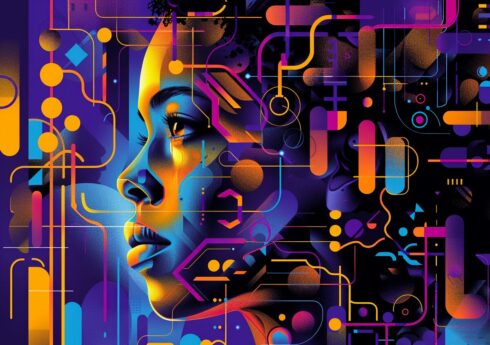
In the world of technology, change is the norm, and the adoption of cutting-edge solutions is not just a matter of staying competitive but of survival. Today’s leading organizations rely on a complex tapestry of data centers, public cloud and SaaS providers, traditional network and security devices, physical and virtual servers, and a blend of legacy applications and the latest containerized resources.
Not only has change become the norm, but so has complexity. This complexity means that IT needs a new and better way to keep the business running.
The impact of generative AI and large language models will be profound for IT operations and business leaders. Generative AI and LLMs like the GPT series by OpenAI and BERT by Google are transforming observability into a more efficient, accessible, and democratized practice. They’re reshaping the landscape for network engineers, cloud professionals, DevOps, SREs, and both technical and business leaders.
The Impact of Generative AI on Observability
Simplifying Complexity with Generative AI
The core strength of generative AI is its ability to process and make sense of vast amounts of data faster and more accurately than any human can. Observability in technical fields involves the daunting task of parsing immense datasets of documentation, telemetry, code, business information, etc. It involves understanding very complex systems and drawing actionable insights – this is where generative AI and LLMs make a huge difference.
For example, generative AI models in network engineering can process real-time traffic data and quickly identify patterns and anomalies that might indicate potential failures or security threats. Normally, this activity would require a team of engineers, an entire suite of tools, and likely a significant amount of time.
Similarly, in cloud engineering, LLMs can analyze cloud server logs, performance metrics, and cost trends to pinpoint inefficiencies in hybrid or multi-cloud activity, a difficult task for even the most adept IT professionals.
The ability of these models to turn complex data into understandable insights helps with better and faster decision-making and reduces the reliance on specialized skills for data interpretation.
Enhancing Capacity Planning and Predictive Analysis
Capacity planning is another critical aspect of ensuring resource efficiency and reliable operational performance. Generative AI excels in leveraging historical data to identify trends and patterns to forecast future needs. For example, generative AI models can analyze past usage patterns in a cloud environment to predict upcoming resource requirements, ensuring that the infrastructure scales efficiently without wasteful and expensive over-provisioning.
This means that with a predictive analysis capability, AI workflows can identify trends that humans would likely miss. By providing insights into potential future scenarios, they enable proactive measures rather than reactive fixes, a typical struggle for most IT teams.
Streamlining Operations for DevOps and SREs
DevOps and Site Reliability Engineers often wrestle with the dual challenge of maintaining system reliability while rapidly delivering new features. LLMs can be instrumental in this space by automating routine tasks like log analysis, monitoring system health, and even suggesting code optimizations or fixes. This not only speeds up the development cycle but also frees up SREs and DevOps engineers to focus on more strategic, high-impact work.
By using LLMs to interface with vast amounts of data, DevOps engineers can also interrogate complex and enormous application data sets faster and with more insight than is possible manually. This benefit alone is a huge step forward in streamlining and improving data analysis, the lifeblood of maintaining successful application delivery.
SREs ensure the reliability, uptime, and performance of an entire complex environment. Generative AI tools can be used to forecast and predict traffic patterns indicating a potential DDoS attack, identify under-provisioned virtual environments that could lead to resource exhaustion, detect incremental but steady cloud cost increases, and detect a myriad of other indicators of potential problems.
Democratizing Information Across Teams
Next, possibly one of the most transformative aspects of generative AI, particularly LLMs, is their role in democratizing technical information. Typically, the deep technical knowledge required for system observability has been confined to a small group of experts or embedded in vast, inaccessible databases.
LLMs change this dynamic by acting as a natural language interface between a person and the entire body of underlying data, whether that be logs, telemetry, configurations, technical documents, or a combination of these data types. LLMs make it easy for anyone of any technical skill level to access and explore this data. This allows technical teams and business leaders to have a shared understanding of the system’s state. For business leaders, LLMs help align technical actions with business objectives to facilitate more informed decision-making.
The Future of Generative AI in Observability
Integrating generative AI and LLMs into observability practices marks a significant shift in how businesses manage and interact with their digital infrastructure. Generative AI optimizes current operations and opens doors to innovative approaches in areas like predictive maintenance, advanced cybersecurity, and enhanced user experience monitoring.
For technical and business leaders, integrating AI models into observability strategies is an essential step towards future-proofing their organizations. It promises not just incremental improvements but a fundamental rethinking of how we interact with and leverage our digital resources.
As we explore the possibilities generative AI offers, one thing is clear—the future of technology is not just about managing complexity; it’s about mastering it for our own strategic advantage.