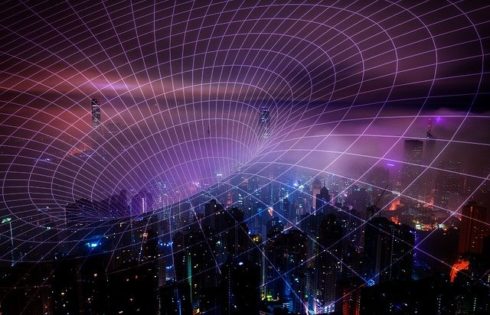
In today’s environment, both within the tech industry and outside, the topic of data growth is a popular one. But the reality is that data isn’t just growing. It’s exploding. In 2010, 1.2 zettabytes of brand new data were created. That’s more than had been created in all of previous human history. This year, humanity is expected to generate upwards of 33 zettabytes. By 2025, the number is expected to be 175 zettabytes. That’s not just exponential growth, it’s the emergence of a completely different asset. This new reality requires a mindshift in the way we collect, store and analyze information that’s already at our disposal. Brand new industries are being built around this reality, while others that have been around for decades struggle to adjust to the new normal.
Given what’s predicted to come down the pike, we really haven’t even scratched the surface of what data can do for business — or, its potential to doom unprepared organizations and industries. In the automotive space, every car manufacturer is focused on self-driving vehicles. This endeavor represents an entirely new model of how to collect, manage and process data. Suddenly, we have individual companies generating data at the 100 petabyte level, all competing to build the best product in a brand new market. In telecommunications, 5G has brought speeds and latency much closer to high-speed networking available at the edge. To build that out requires a ton of infrastructure. In healthcare, imaging, genome sequencing and drug discovery have created an explosion of data and an opportunity to revolutionize the way we practice medicine. This is work that cannot and should not be held back by old-world tech hiding underneath. Future success is dependent on a fresh approach to the infrastructure layer.
Modern applications and modern data, unsurprisingly, come equipped with a constantly evolving set of challenges. Those challenges break down into what are called “workloads” — a series of tasks required from infrastructure to successfully run an application and glean value from the results. At the core of any organization’s infrastructure is storage. Modern data needs to be stored in a way that it is accessible so that it can be turned into value, retains its integrity so that it can supply historical insight and future predictions, and in a way that accounts for expected — and unexpected — scale. The ability to consolidate these workloads into a single platform is critical in today’s fast-paced business world.
Previous file or object storage systems were built specifically for one function or the other, and how that function managed metadata. File was built on hierarchical systems, while object was designed to store at massive scale through slow access methods. Today, that simply doesn’t cut it. But building a database that has rich metadata capabilities, rich enough to layer both file and object semantics on top like tents of an underlying platform is what’s required. This fundamental database approach under the covers has never been done before, yet demand for this approach is growing exponentially, and organizations are hard-pressed to find solutions that are organically engineered to meet it. As organizations continue along their (now accelerated) journey to digital transformation and begin to realize their structural goals, it quickly becomes clear that performance and simplicity at scale requires a unified approach.
Let’s start on familiar ground — data protection. Protecting the integrity and viability of data, today’s most valuable industrial currency, is paramount for any and all modern organizations. Data protection is a team effort between file and object storage. The standard architecture that we’ve built in partnership with Commvault, for example, relies on a combination of file and object storage for deployment. While parts of the architecture need a File API, the bulk data is object. Unified Fast File and Object (UFFO) allows for both these tasks to run on a single platform, which simplifies management, reduces cost and puts fewer vendors between a customer and their data.
Then there’s the other side of this same coin — the need to keep data safe from malicious actors, attack or theft. The average consumer would be bewildered by the scale of what a modern retailer, for example, has to do to ensure security through the explosive growth of telemetry data. Apps deployed for the business and the transition from physical to online sales leave modern organizations more vulnerable, and digital transformation has exponentially expanded the cyberattack surface. This problem requires organizations to peer around corners, to protect what they can’t necessarily even see. Organizations prevent future attacks by identifying and running analysis on attacks that have already happened. Naturally, this entails the collection of enormous amounts of telemetry log data and forensic information.
For example, consider an organization in the financial industry that has been in the business of fraud detection since its inception. Every year, attacks and fraud become more sophisticated. Every time one angle is shut down, the malicious actors evolve and move to another. What may surprise you is their team’s use of a unified file and object storage platform to facilitate machine learning to build state of the art fraud detection models. This process looks a little bit like software development – which requires an object store – while the output of machine learning tools all pretty much run on file. Even at companies of massive scale, file is preferable because researchers tend to prototype on their laptops and want the same familiar abstractions at scale.
There is also a critical need emerging for a unified approach to file and object storage in the area of quantitative finance. Their dominant workload is a virtual firehose of unstructured data — market tick data, stock feeds, options feeds, anything that can be processed to help a financial model make more accurate predictive decisions. IoT companies face this same problem with the continued explosion of connected devices, but in the finance world they’ve been dealing with this issue for decades. They tend to utilize data stores that need file under the hood.
Today, every organization needs to establish a top-down approach to building a true internal data platform, where information is analyzed and curated so that downstream consumers gain value from that data. evolve with their needs, which may be ever in-process or can even shift overnight.